Fair Machine Learning & AI
Machine Learning and AI are taking off, also in higher education. These are valuable methods to use for analysing study data. But how fair and (un)biased are the resulting data and analyses?
Centre of Expertise Global and Inclusive Learning
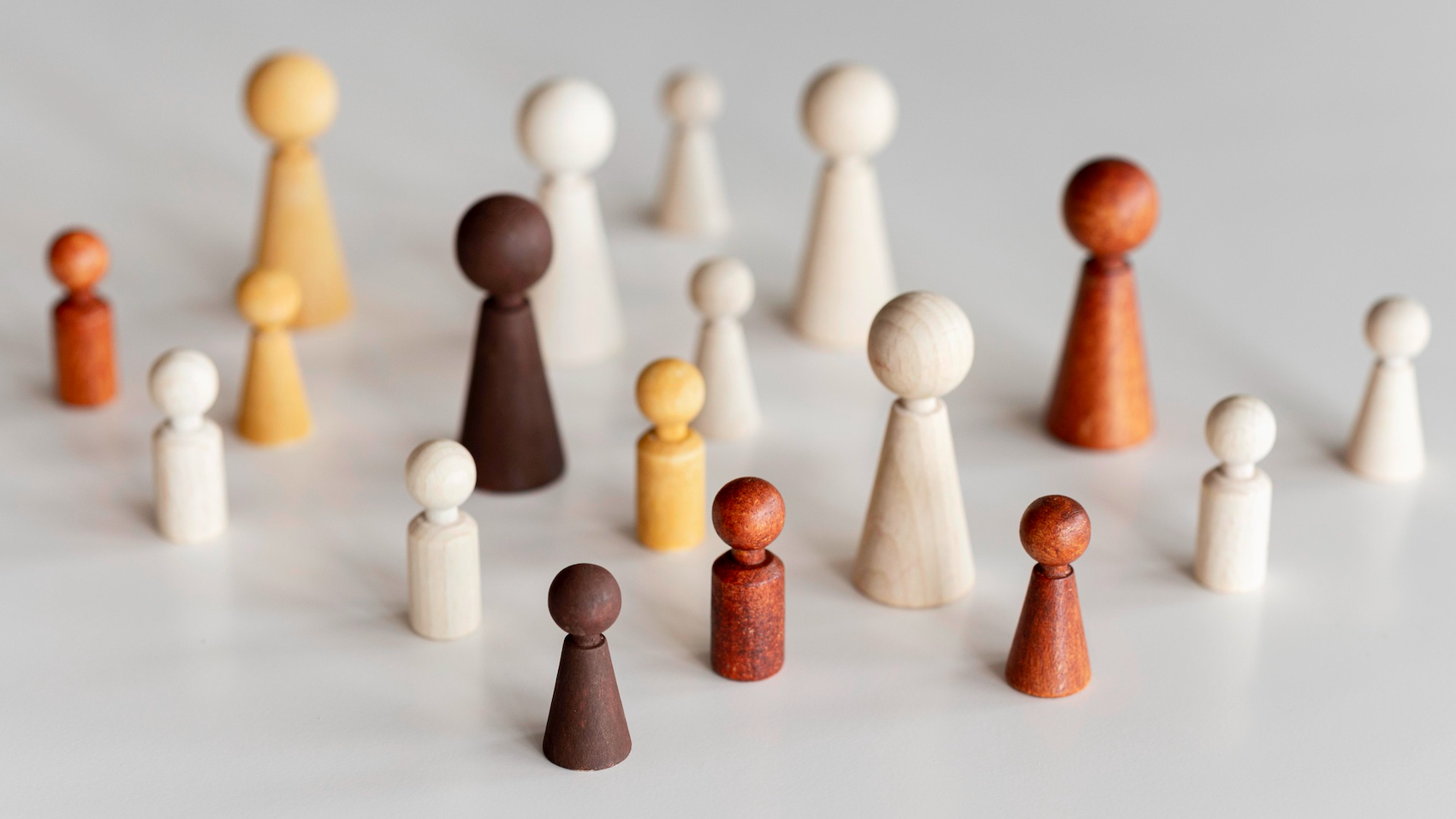
Machine Learning and AI are taking off, also in higher education. These are valuable methods to use for analysing study data. But how fair and (un)biased are the resulting data and analyses?
Within the Learning Technology & Analytics research group, we research fairness and bias concerning Machine Learning and AI in higher education.
Fairness is relevant in several ways: Is data collection fair? Are we doing enough justice to the students whose study data we examine? What do study data tell us about how fair we act as educational institutions? Are algorithms fair? Is using prediction models by teachers, supervisors, or policy staff fair?
Balanced
An example of this type of research is the PhD research of Professor Theo Bakker. In it, he used a statistical method, propensity score weighting, to balance the data of students with and without autism. This technique helps us better understand the differences between groups and enables us to adjust guidance and policy accordingly.
Balanced research also helps to re-evaluate biases. For example, the study, after balancing study data of students with autism, found that their study progress was almost as good as that of their peers but also showed that better preparation for testing was a focus point. This kind of correction to data should be a standard approach when examining (minority) groups of students in higher education.
Plans
Other questions we want to answer within this project over time are:
- Which biases occur at which place in the data science process?
- What examples can we find from educational practice?
- Which concepts of fairness exist?
- What can we do to reduce bias and increase fairness?
- What are the benefits and trade-offs?
Contact
Are you interested in the outcomes of this study, or would your study programme like to participate? If so, please contact Professor Theo Bakker at [email protected].